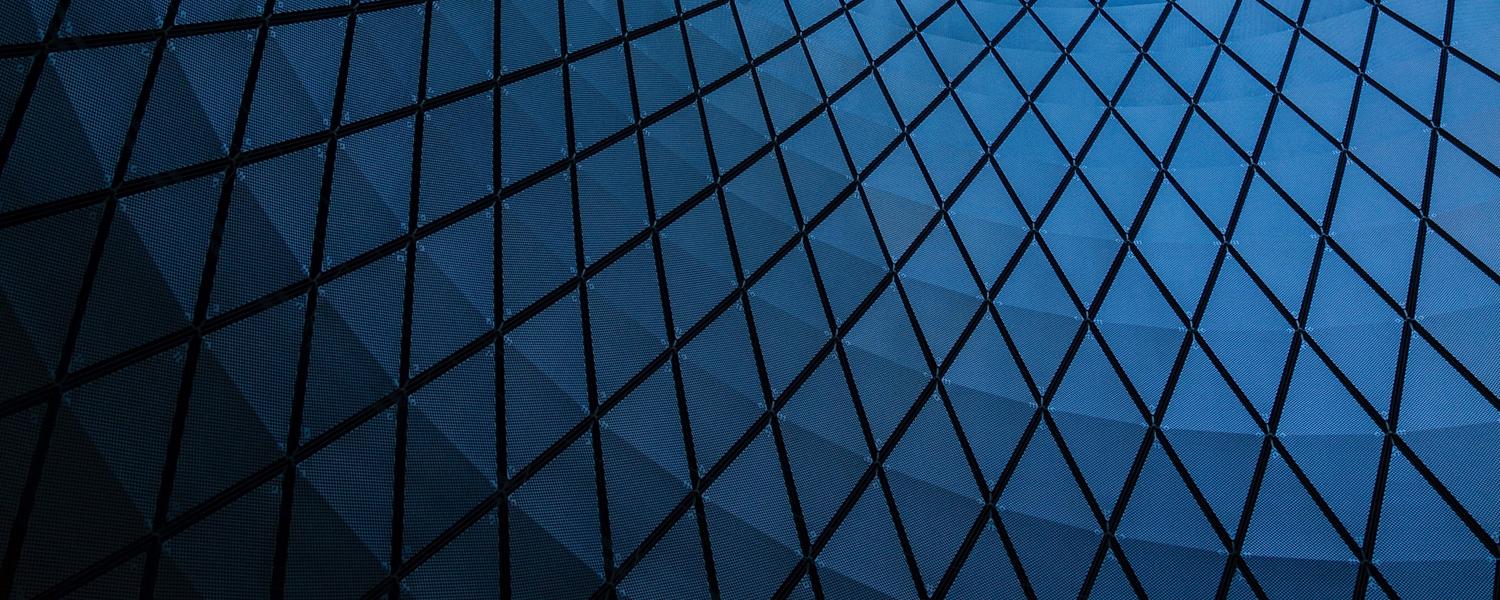
Generative Modeling
MIPLab's research with generative modeling
We are developing novel statistically-motivated machine learning approaches to analyze and model shape and appearance variations of anatomical and pathological structures (e.g., organs) at a subject-specific or population level based on medical images. Those models then serve as predictors in clinical practice, exploratory tools in research, or prior information within advanced medical image analysis tools. Our applications are especially focused on modeling and analyzing normal and degenerative processes in the human brain (e.g., brain aging, Alzheimer’s, and Parkinson’s) but also encompass related scenarios from other subdomains of medical imaging like facial shape analysis. In addition to these application-specific projects, we also develop more general machine learning strategies to allow for generative modeling in scenarios where medical image data for model training is typically scarce or hard to obtain.
Shape modeling with few training data
Generative statistical shape models have a long and successful history in medical image analysis as priors for image segmentation or registration tasks. We develop strategies to allow their use in scenarios where training data is limited and standard models fail to generalize.
Modeling and analysis of brain aging
Aging leads to considerable morphological changes of the human brain and image-based machine learning approaches can be used to analyze them. Within this context, we develop novel brain age prediction approaches and generative methods to systematically analyze morphological changes.
Facial morphology models for genetic syndrome diagnosis
Many human genetic syndromes result in subtle facial differences that are hard to distinguish. We develop automated machine learning tools that help clinicians and researchers to identify and analyze genetic syndromes based on facial shape scans.