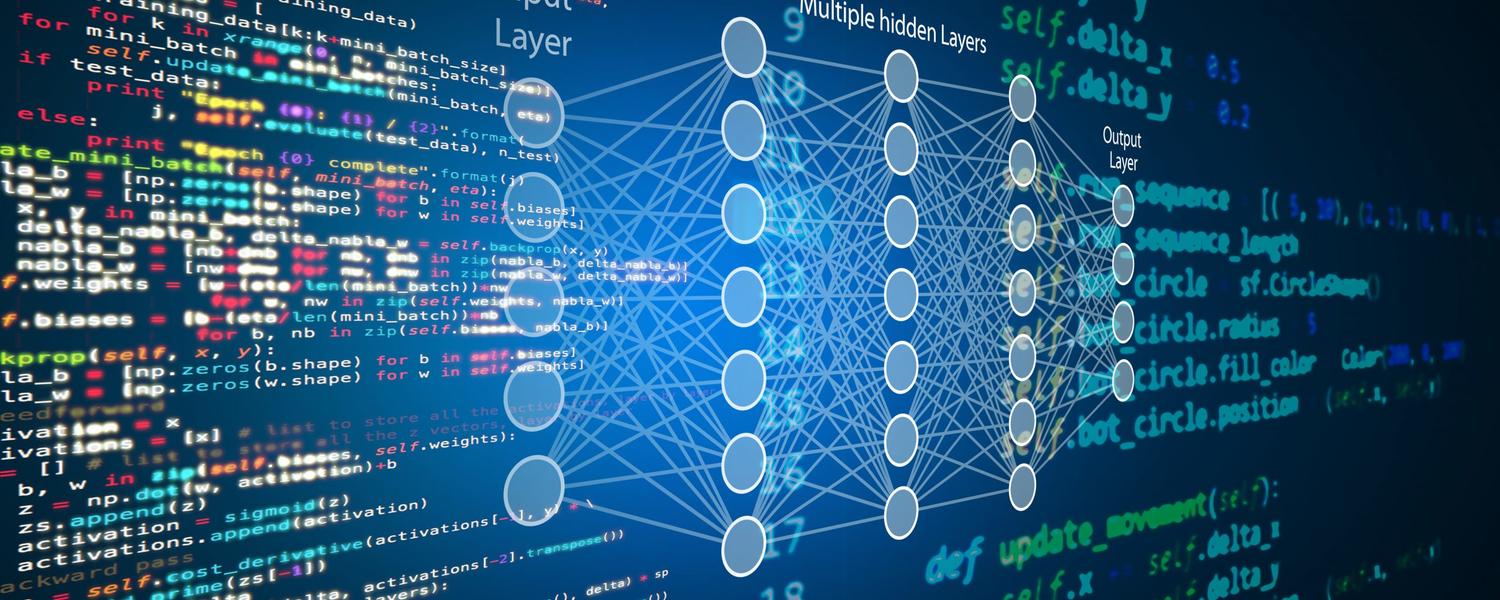
Predictive analytics and diagnostic support
Medical datasets often contain mixed and incomplete data including multi-modality imaging features, clinical tests, lifestyle factors, and demographic information. Given the complexity of such data, machine learning methods are a useful and often necessary tool for providing high-quality predictive analytics and diagnostic support. Within the scope of machine learning, however, the construction of a model requires myriad design decisions, each with their own implications for the interpretability, robustness and clinical relevance of the resulting analysis.
In these projects, our aim is to describe and evaluate key model design decisions in a diverse set of clinical contexts included but not limited to ischemic stroke. The long-term goal for this line of investigation is to provide clinicians with model designs that can be used in accurate and reliable decision support frameworks.
Stroke tissue outcome prediction
Predicting the tissue outcome in an acute ischemic stroke is essential for therapy decision-making since each treatment has its own inherent risks. Therefore, our current research in this area focuses on developing treatment-specific prediction models for estimating the final tissue outcome using high-level machine learning techniques.
Stroke clinical outcome prediction
Functional recovery following stroke is a complex phenomenon that depends on both neuroanatomical and clinical factors. Using statistical and machine learning approaches, we are developing models to identify relevant variables for predicting patients’ long-term clinical outcomes.
Model selection for applied machine learning research
Using an in-house automated machine-learning pipeline, we support researchers in the Hotchkiss Brain Institute and the Alberta Children’s Hospital to conduct meta-analyses of their datasets for a given pathology.