Causal Machine Learning
Causal reasoning can offer fresh insights into the primary challenges within the field of medical imaging in machine learning: explainability, the limited availability of annotated data, and the disparity between the training dataset and the target environment.
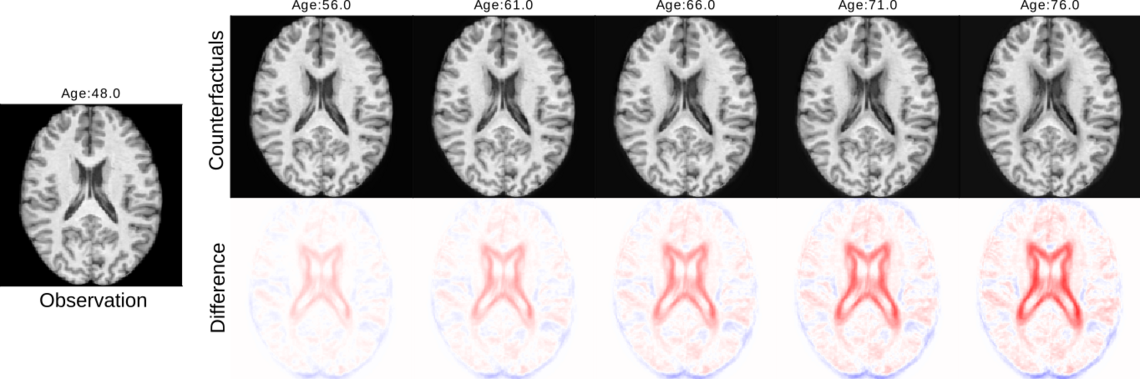
Causal analysis of the relationships between the brain and cardiovascular risk factors
Previous studies have reported that risk factors for cardiometabolic diseases are associated with accelerated brain aging. However, these studies were primarily based on standard correlation analyses, which do not unveil a causal relationship. While randomized controlled trials are typically required to investigate true causality, we investigate an alternative method by exploring data-driven causal discovery and inference techniques on observational data.
Harmonizing neuroimaging data with counterfactual inference
Deep learning has led to many advances in medical image analysis for various clinical problems. However, most deep learning models are known to be sensitive to differences in the training and test data distributions, which can lead to a decrease in accuracy when applied in real-life scenarios. Thus far, various techniques have been developed to tackle this problem, primarily focusing on harmonizing feature representations from different datasets. Due to the recent increased interest in causal approaches in deep learning, explainable harmonization techniques have gained momentum lately but have not been applied broadly yet. This project proposes a causal flow-based technique to overcome the problem of different feature distributions in multi-site data.
Integrating causal structures into generative models with masked causal flow (MACAW)
While deep learning techniques show promising results in neuroimaging tasks on theoretical grounds, they have not yet found widespread use in clinical settings. One of the reasons behind this is the inherent complexity and lack of transparency associated with these models. To tackle this issue, numerous explainable AI (XAI) techniques have been developed. However, many of these methods are primarily post-hoc, which might not fully capture the model's actual behavior. This project focuses on developing a novel deep learning architecture called Masked Causal Flow (MACAW), which combines the advancements of Causal AI and generative modelling.