Downloads
Download our publicly available resources and code.
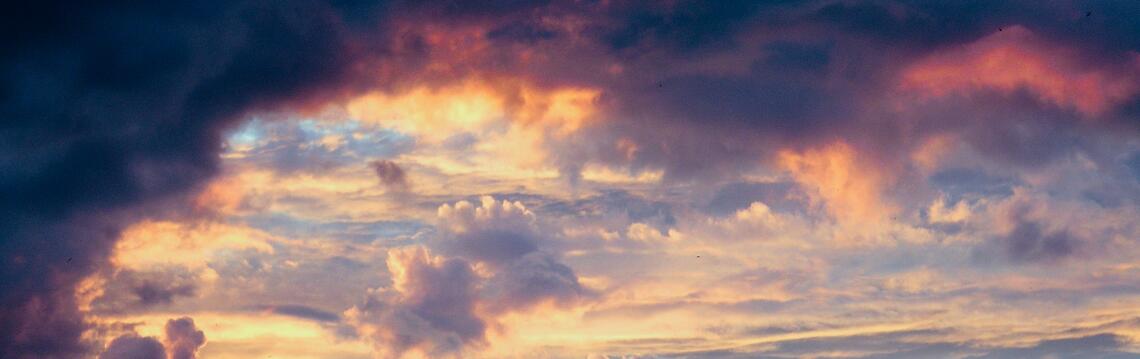
FLAIR and NCCT atlases for the elderly
Normative brain atlases are a standard tool for neuroscience research and are, for example, used for spatial normalization of image datasets prior to voxel-based analyses of brain morphology and function. Although many different atlases are publicly available, they are usually biased with respect to an imaging modality and the age distribution. Both effects are well known to negatively impact the accuracy and reliability of the spatial normalization process using non-linear image registration methods. An important and very active neuroscience area that lacks appropriate atlases is lesion-related research in elderly populations (e.g. stroke, multiple sclerosis) for which FLAIR MRI and non-contrast CT are often the clinical imaging modalities of choice. To overcome the lack of atlases for these tasks and modalities, this paper presents high-resolution, age-specific FLAIR and non-contrast CT atlases of the elderly built from clinical images.
We provide an asymmetric and symmetric version of both the FLAIR and NCCT atlases in the standard MNI coordinate system with binary masks for the brain volume. We also provide the deformation field to map parcellations in the MNI space to our atlases (both symmetric and asymmetric) should researchers require this in their individual research projects.
FLAIR atlas NCCT atlas Deformation filesPublication:
Deepthi Rajashekar, Matthias Wilms, M. Ethan MacDonald, Jan Ehrhardt, Pauline Mouches, Richard Frayne, Michael D. Hill, and Nils D. Forkert.
High-resolution normative T2-FLAIR and CT brain atlas of the elderly
Scientific Data, 7, 56, 2020.
URL: https://www.nature.com/articles/s41597-020-0379-
9
A statistical atlas of cerebral arteries
Magnetic resonance angiography (MRA) can capture the variation of cerebral arteries with high spatial resolution. These measurements include valuable information about the morphology, geometry, and density of brain arteries, which may be useful to identify risk factors for cerebrovascular and neurological diseases at an early time point. However, this requires knowledge about the distribution and morphology of vessels in healthy subjects. the statistical arterial brain atlas described in this work is a free and public neuroimaging resource that can be used to identify vascular morphological changes. The atlas was generated based on 544 freely available multi-center MRA and T1-weighted MRI datasets. the arteries were automatically segmented in each MRA dataset and used for vessel radius quantification. The binary segmentation and vessel size information were non-linearly registered to the MNI brain atlas using the T1-weighted MRI datasets to construct atlases of artery occurrence probability, mean artery radius, and artery radius standard deviation. This public neuroimaging resource improves the understanding of the distribution and size of arteries in the healthy human brain.
The statistical atlas consists of four image files in the niftii format and in MNI reference space (0.5 mm^3). These files include the TOF MRA average atlas (tofAverage.nii.gz), the vessel probability atlas (vesselProbabilities.nii.gz [in %]), the mean artery radius atlas (vesselRadius.nii.gz [in mm]), and the standard deviation of the artery radius atlas (vesselRadiusStd.nii.gz [in mm]). All images are saved using float values. The TOF MRA and T1-weighted datasets used for atlas generation can be downloaded from the original sources.
Time of flight atlas Probabilistic vessel atlas Radius vessel atlas (average) Radius vessel atlas (standard deviation)Publication:
Mouches, Pauline, and Nils D. Forkert.
A statistical atlas of cerebral arteries generated using multi-center MRA datasets from healthy subjects.
Scientific Data, 6(1), 29, 2019.
URL: https://www.nature.com/articles/s41597-019-0034-5
Automatic segmentation of stroke lesions in non-contrast computed tomography datasets with conv...
Non-contrast computed tomography (NCCT) is commonly used for volumetric follow-up assessment of ischemic strokes. However, manual lesion segmentation is time-consuming and subject to high inter-observer variability. The aim of this study was to develop and establish a baseline convolutional neural network (CNN) model for automatic NCCT lesion segmentation. A total of 252 multi center clinical NCCT datasets, acquired from 22 centers, and corresponding manual segmentations were used to train (204 datasets) and validate (48 datasets) a 3D multi scale CNN model for lesion segmentation. Post processing methods were implemented to improve the CNN-based lesion segmentations. The final CNN model and post processing method was evaluated using 39 out of distribution holdout test datasets, acquired at seven centers that did not contribute to the training or validation datasets. Each test image was segmented by two or three neuroradiologists. The Dice similarity coefficient (DSC) and predicted lesion volumes were used to evaluate the segmentations. The CNN model achieved a mean DSC score of 0.47 on the validation NCCT datasets. Post-processing significantly improved the DSC to 0.50 (P<0.01). On the holdout test set, the CNN model achieved a mean DSC score of 0.42, which was also significantly improved to 0.45 (P<0.05) by post processing. Importantly, the automatically segmented lesion volumes were not significantly different from the lesion volumes determined by the expert observers (P>0.05) and showed excellent agreement with manual lesion segmentation volumes (intraclass correlation coefficient, ICC = 0.88). The proposed CNN model can automatically and reliably segment ischemic stroke lesions in clinical NCCT datasets. Post processing techniques can further improve accuracy. As the model was trained and evaluated on datasets from multiple centers, it is broadly applicable and is publicly available.
Publication:
Anup Tuladhar*, Serena Schimert*, Deepthi Rajashekar, Helge C. Kniep, Jens Fiehler, Nils D. Forkert, "Automatic Segmentation of Stroke Lesions in Non-Contrast Computed Tomography Datasets With Convolutional Neural Networks," in IEEE Access, vol. 8, pp. 94871-94879, 2020, doi:10.1109/ACCESS.2020.2995632. *: Co-first authors