Modeling and analysis of brain aging
Aging results in morphological changes of the human brain and machine learning can help to systematically analyze and predict them using structural MR images. Our research focuses on novel machine learning techniques that can be used for biological brain age estimation, the generation of age-specific average brain morphology templates that realistically represent the aging effects, the systematic analysis of morphological variations, and that allow for subject-specific brain aging simulation. To this end, we are specifically interested in developing approaches that unify brain age estimation and generative modeling with cutting edge machine learning techniques like normalizing flows. We also work on the integration of non-imaging clinical and lifestyle factors like gender and weight that allow for the detection of novel biomarkers for aging-related neurodegenerative diseases.
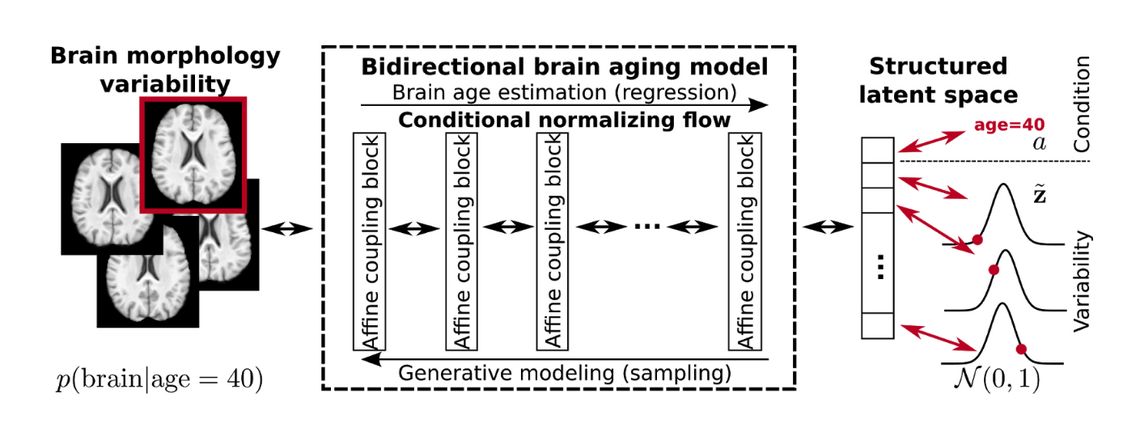
Publications
Matthias Wilms, Jordan J. Bannister, Pauline Mouches, M. Ethan MacDonald, Deepthi Rajashekar, Sönke Langner, Nils D. Forkert: Bidirectional modeling and analysis of brain aging with normalizing flows, Machine Learning in Clinical Neuroimaging Workshop, held in conjunction with the 23rd International Conference on Medical Image Computing and Computer Assisted Intervention, Lima, Peru, 2020. https://arxiv.org/pdf/2011.13484
Pauline Mouches, Banafshe Felfeliyan, Sönke Langner, Nils D. Forkert: Biological brain age prediction ability of different subcortical structures using deep learning, 26th Annual Meeting of the Organization for Human Brain Mapping, Montreal, Canada, 2020.
Deepthi Rajashekar, Matthias Wilms, M. Ethan MacDonald, Jan Ehrhardt, Pauline Mouches, Richard Frayne, Michael D. Hill, Nils D. Forkert: High-resolution normative T2-FLAIR and CT brain atlas of the elderly, Scientific Data, 7, 56, 2020.
Team members
-
Jordan J. Bannister
-
Nils D. Forkert
-
Pauline Mouches
-
Deepthi Rajashekar
-
Matthias Wilms