Shape modeling with few training data
Generative statistical shape models have a long and successful history in medical image analysis as priors for image segmentation or registration tasks. Nowadays they are also successfully utilized within deep learning approaches where they either serve as strong prior knowledge or are being used during model training to generate additional artificial but realistic training data. A key problem of traditional statistical shape models is their inability to generalize well to unseen new data when they were trained with few samples. Resulting models will mostly focus on global shape relations and not be able to model the true variability to be expected in a large population. To alleviate this problem, we develop shape modeling approaches that use data-driven and physics-inspired mechanisms during training to create more flexible models even in small training data regimes. Key contributions are the introduction of the principle of locality, which breaks up long-range relations in the training data, and kernel-based techniques to allow the integration of this principle into medical image analysis frameworks that use large 3D data.

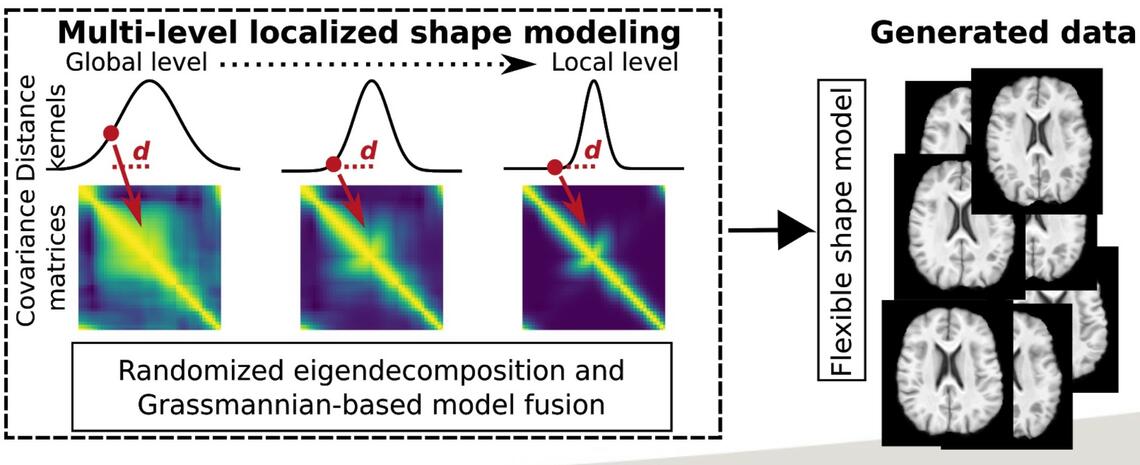
Publications
Matthias Wilms, Jan Ehrhardt, Nils D. Forkert: A kernelized multi-level localization method for flexible shape modeling with few training data In: Martel A.L. et al. (eds) Medical Image Computing and Computer Assisted Intervention – MICCAI 2020. MICCAI 2020. Lecture Notes in Computer Science, Vol 12264, 765-775, 2020. Github: https://github.com/wilmsm/localizedssm
Hristina Uzunova, Paul Kaftan, Matthias Wilms, Nils D. Forkert, Heinz Handels, Jan Ehrhardt: Quantitative comparison of generative shape models for medical images In: Tolxdorff T. Deserno T.M., Handels H., Maier A., Maier-Hein K.H., Palm C. (eds.), Bildverarbeitung für die Medizin 2020, Heidelberg, Informatik aktuell, Springer Vieweg, Berlin Heidelberg, 201-207, 2020.
Team members
-
Nils D. Forkert
-
Matthias Wilms