Decoding Rocks: A Physics-Driven Framework for Predicting Subsurface Properties
Understanding how heat moves through porous rocks is critical for subsurface applications like geothermal energy, reservoir modeling, and thermal conductivity logging. Our research introduces a powerful framework that combines hierarchical homogenization, graph-based machine learning, and cross-property physics models to predict thermal and elastic properties from Micro-CT images, quickly and accurately.
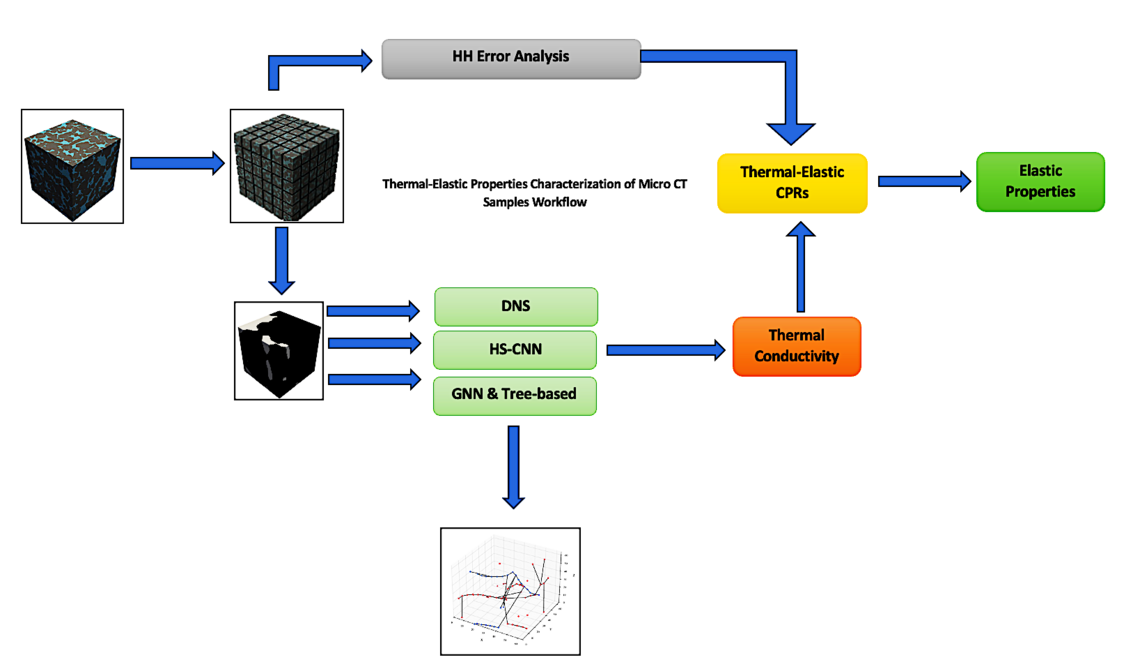
Key Takeaways
- Identified error sources in thermal conductivity calculations using hierarchical homogenization, especially related to sample heterogeneity
- Achieved up to R² = 0.99 using GNNs and physics-informed CNNs trained on Micro-CT-based graphs
- Developed cross-property correlations linking thermal conductivity and elasticity, enabling accurate estimation of one from the other in both dry and saturated conditions
Why It Matters
These tools reduce reliance on time-consuming lab tests, enhance thermal conductivity logs with inferred elastic data (and vice versa), and improve modeling in data-sparse environments. Especially in geothermal and carbon storage operations, this smarter, faster approach to rock property estimation supports better design, monitoring, and decision-making.
We're making rock physics smarter—where physics meets data, and innovation meets impact.